Rethinking Enterprise LLM: Secure, Cost-Effective AI

As AI becomes central to business innovation, the decision between cloud-based and on-premise Large Language Models (LLMs) is more critical than ever. If you're exploring ways to leverage enterprise LLMs without compromising on data security or cost control, you're in the right place.
In this guide, I’ll walk through how deploying LLMs locally offers distinct advantages, from heightened data confidentiality to budget-friendly AI solutions. By the end, you’ll see why more organizations are moving their AI in-house and how you, too, can make your enterprise LLM deployment both secure and efficient. Let’s get started!
Introduction to Enterprise LLM: Trends and Challenges
In recent years, enterprise large language models (LLMs) have gained traction, revolutionizing how organizations interact with and interpret data. Typically, enterprises rely on cloud-based LLMs, which offer scalability and immediate accessibility. However, as data security concerns rise, more organizations are considering local language models to keep data closer to home. This shift towards on-premise LLM deployments reflects a growing demand for privacy, control, and compliance, especially within regulated industries such as healthcare, finance, and law.

Let’s explore how rethinking cloud-based LLM strategies for local deployment can address these emerging challenges.
Why Local Language Models Are Gaining Popularity
The appeal of local language models lies in their ability to address key enterprise needs for data confidentiality and control. Unlike cloud-hosted LLMs, which require data to be sent offsite, on-premise models allow organizations to host AI solutions directly within their infrastructure. This capability is essential for sectors that handle sensitive or proprietary information.

On-premise LLM deployments enable businesses to comply with stringent regulatory standards, as data is processed and stored on-site, limiting exposure to external networks. By prioritizing enterprise data confidentiality, organizations can benefit from AI without compromising on privacy.
RAG in Enterprise LLMs: Enhancing Accuracy and Efficiency
A key advantage of deploying on-premise LLMs is the potential to integrate Retrieval-Augmented Generation (RAG) techniques, enhancing the AI's ability to provide precise, contextually relevant responses. RAG combines language models with a retrieval system, allowing enterprises to pull data from specific, reliable sources in real time. This method is especially useful in data-sensitive sectors, where LLMs can generate responses informed by up-to-date, secure information stored locally.
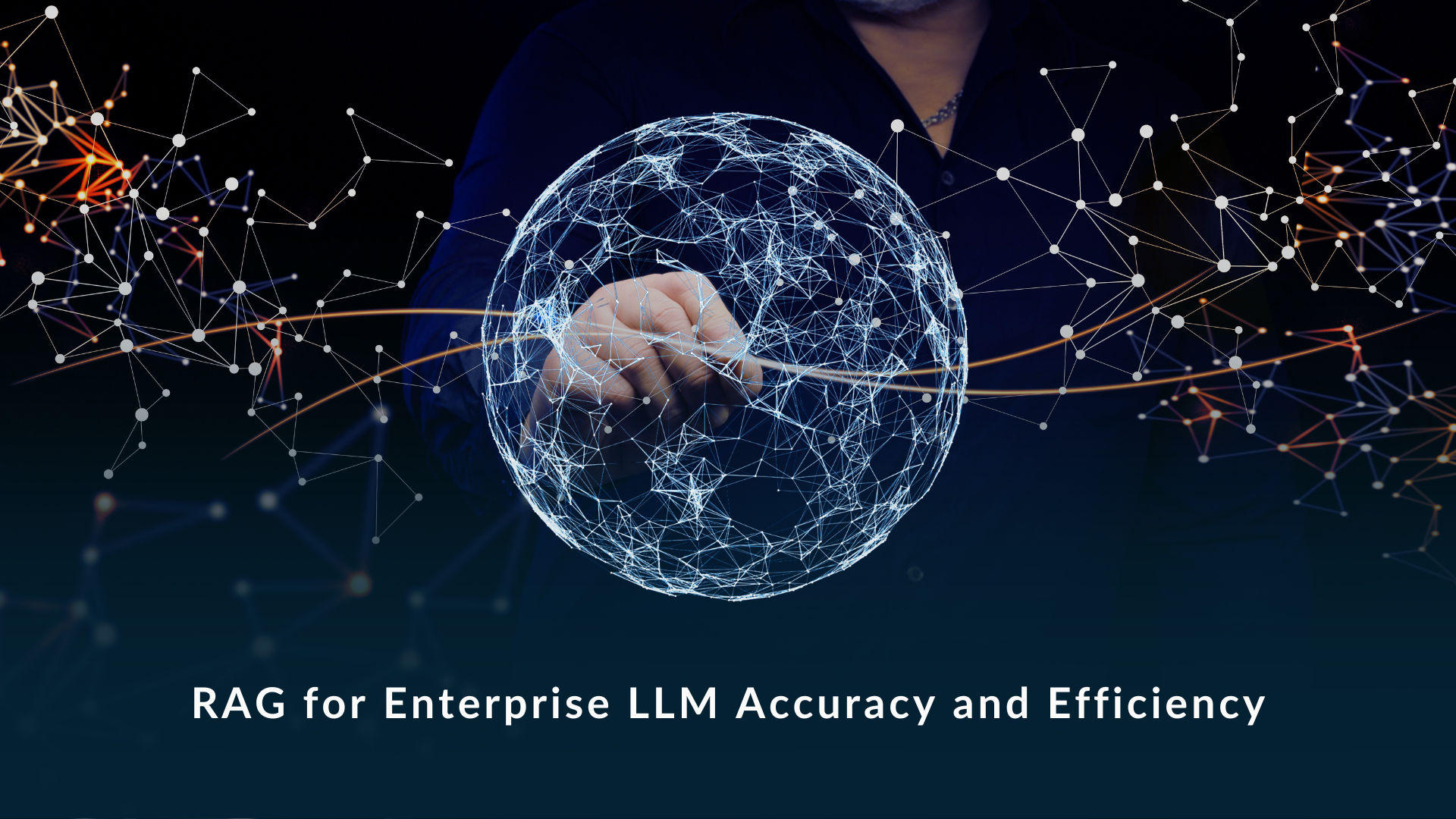
With RAG, organizations using QAnswer’s on-premise LLM solutions can improve response accuracy while ensuring data remains confidential and compliant with internal standards. This fusion of retrieval and generation further strengthens the appeal of local language models for enterprises aiming for both enhanced performance and robust data privacy.
The Security Advantage of On-Premise LLMs
One of the foremost benefits of on-premise LLM deployments is the heightened level of security they offer. Cloud-based LLMs can be vulnerable to data breaches, as sensitive information must travel to and from third-party servers. With on-premise LLMs, organizations retain exclusive control over their data. This is especially valuable in sectors where data privacy and enterprise data confidentiality are non-negotiable.

At The QA Company, our QAnswer platform supports secure configurations that keep all data interactions within an organization’s own firewall, minimizing risks associated with third-party access and enabling compliance with internal data protection policies.
Performance of On-Premise LLMs vs. Cloud-Based Solutions
Contrary to the assumption that cloud-based models are inherently superior, on-premise LLMs can offer performance levels comparable to, and sometimes exceeding, their cloud counterparts. By running models within their own networks, enterprises can optimize local language model performance according to their infrastructure specifications.

With QAnswer, enterprises gain access to a range of LLMs that they can configure and test within the same platform. This flexibility makes it easy to compare the QAnswer LLM, with popular cloud-based LLMs like OpenAI LLM and Mistral LLM. Such comparisons empower businesses to tailor performance to their unique needs.
Cost Management Benefits with Local LLM Deployments
Switching to on-premise LLM deployments isn’t just about security and data control—it’s also a cost-effective choice. Cloud LLMs often come with recurring subscription fees, which can add up significantly over time, especially with large-scale data needs. With local language models, enterprises can avoid these ongoing costs. By making a one-time infrastructure investment, organizations gain budget predictability and cost savings over the long term.

QAnswer’s on-premise LLM options enable companies to maintain budget stability while achieving high-quality AI performance without additional cloud fees.
QAnswer’s Versatile LLM Deployment Options
QAnswer’s platform brings unique flexibility to enterprise LLM deployments. It supports multiple LLMs, enabling enterprises to easily switch between different models, whether they’re open-source or commercially available. For example, companies can test the QAnswer LLM alongside popular models like OpenAI LLM or Mistral, without needing to leave the platform.

This versatility allows organizations to experiment, optimize, and customize large language models according to specific business needs. By providing a single platform for LLM management, QAnswer makes model experimentation accessible and simple.
Industries Benefiting from Local LLM Deployments
Certain industries, such as healthcare, finance, public sectors and law have stringent requirements around data security and enterprise data confidentiality. These sectors stand to benefit significantly from local LLM deployments, where sensitive data never leaves the organization’s infrastructure.

In healthcare, for example, patient data can remain secure within the facility while benefiting from AI-powered diagnostics and information retrieval. Similarly, financial firms can ensure customer data remains private while using LLMs for risk assessment or fraud detection. Legal firms can leverage on-premise LLMs to analyze large volumes of law-case without compromising client confidentiality.
Comparing QAnswer on-premise LLM with other Cloud based models
Gain deeper insights with a comparative study of today’s most promising and widely-used large language models (LLMs).

Key Takeaways - Why QAnswer?
QAnswer’s on-premise solution offers enterprises a high level of data security, confidentiality, and compliance control that cloud-based LLMs often cannot. With flexible integration options and lower latency for local processing, QAnswer is ideal for industries prioritizing data privacy and cost predictability.
Here’s a breakdown of the key benefits:
- Enhanced Data Security: Keeps data interactions entirely within an organization’s firewall, minimizing third-party access and safeguarding sensitive information.
- Full Control and Compliance: On-premise deployment supports strict data privacy standards, making it ideal for regulated industries like healthcare, finance, public sectors and law.
- Cost Efficiency: Reduces long-term expenses by eliminating recurring cloud subscription fees, allowing for budget stability through a one-time infrastructure investment.
- Comparable Performance: Delivers performance on par with cloud LLMs, optimized to leverage existing infrastructure for fast, reliable local processing.
- Flexibility with Multiple LLMs: Supports diverse LLMs (QAnswer LLM, OpenAI LLM, Mistral LLM and more), enabling seamless testing, experimentation, and customization within a unified platform.
- Reduced Latency: Local processing provides faster response times, critical for real-time applications and improved user experiences.
Conclusion
The shift toward on-premise LLMs represents a new era in enterprise AI, where data privacy, cost-effectiveness, and performance coexist. By deploying local language models, enterprises gain full control over their data while minimizing third-party risks. With the flexibility offered by QAnswer, organizations can experiment with various large language models, optimizing performance without compromising enterprise data confidentiality.
Start exploring the benefits of on-premise LLMs today and unlock new AI potential within your own infrastructure.
Discover how QAnswer can empower your business with AI. Reach out at info@the-qa-company.com
Visit our website to learn how QAnswer drives business transformation with AI: www.qanswer.ai
Try QAnswer today and experience its capabilities first hand: https://app.qanswer.ai/
Thank you! See you again with new updates.